Article source:Technology Cloud Report
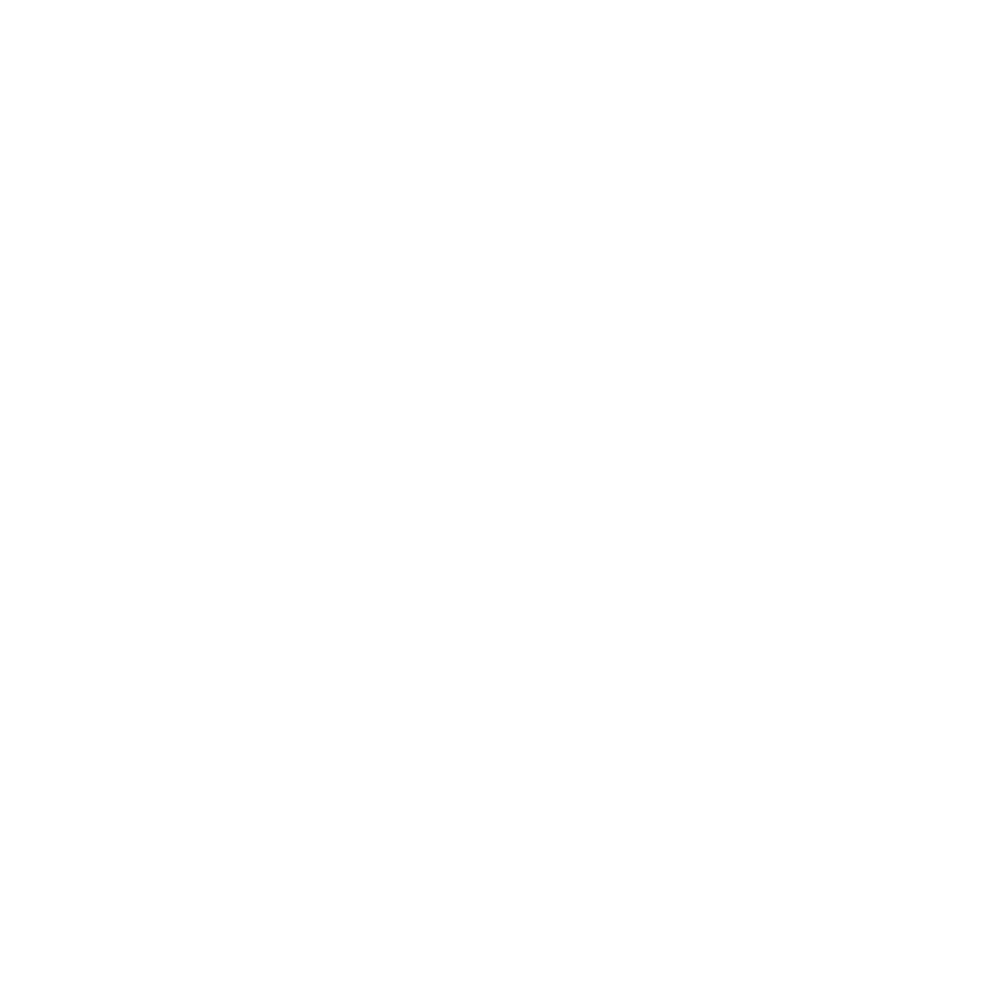
The AI megamodel is forcing the digital infrastructure industry to accelerate its upgrading.
Over the past year and a half, iconic AI big model applications have appeared one after another, from ChatGPT to Sora refreshing people's cognition again and again. Behind the shock is the exponential growth of big model parameters.
The pressure of this data surge is quickly transmitted to the underlying infrastructure of the big model. As the base to support the big model "three big things" - arithmetic power, network, storage, are in rapid iteration.
On the arithmetic side, NVIDIA upgraded its GPUs from H100 to H200 in just two years, giving the models a 5x increase in training performance.
In terms of network, the network bandwidth has been upgraded by 6 times from the previous 25G to the current 200G. With the large-scale application of RDMA, the network latency has also been reduced by 60%.
In terms of storage, Huawei, Aliyun, Baidu Intelligent Cloud, Tencent Cloud and other big players, have launched storage solutions for AI big models one after another.
So what exactly is changing in the AI big model scenario for storage, one of the three main components of infrastructure? And what are the new technical challenges?
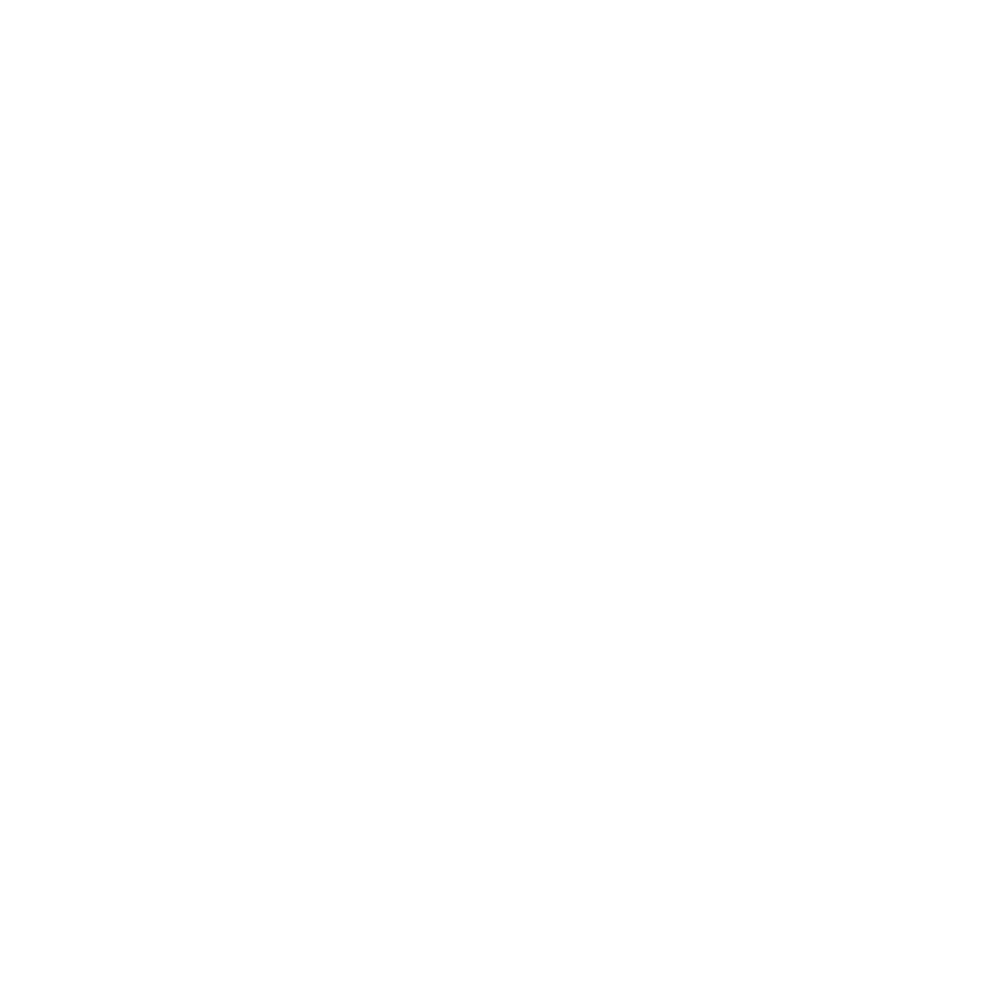
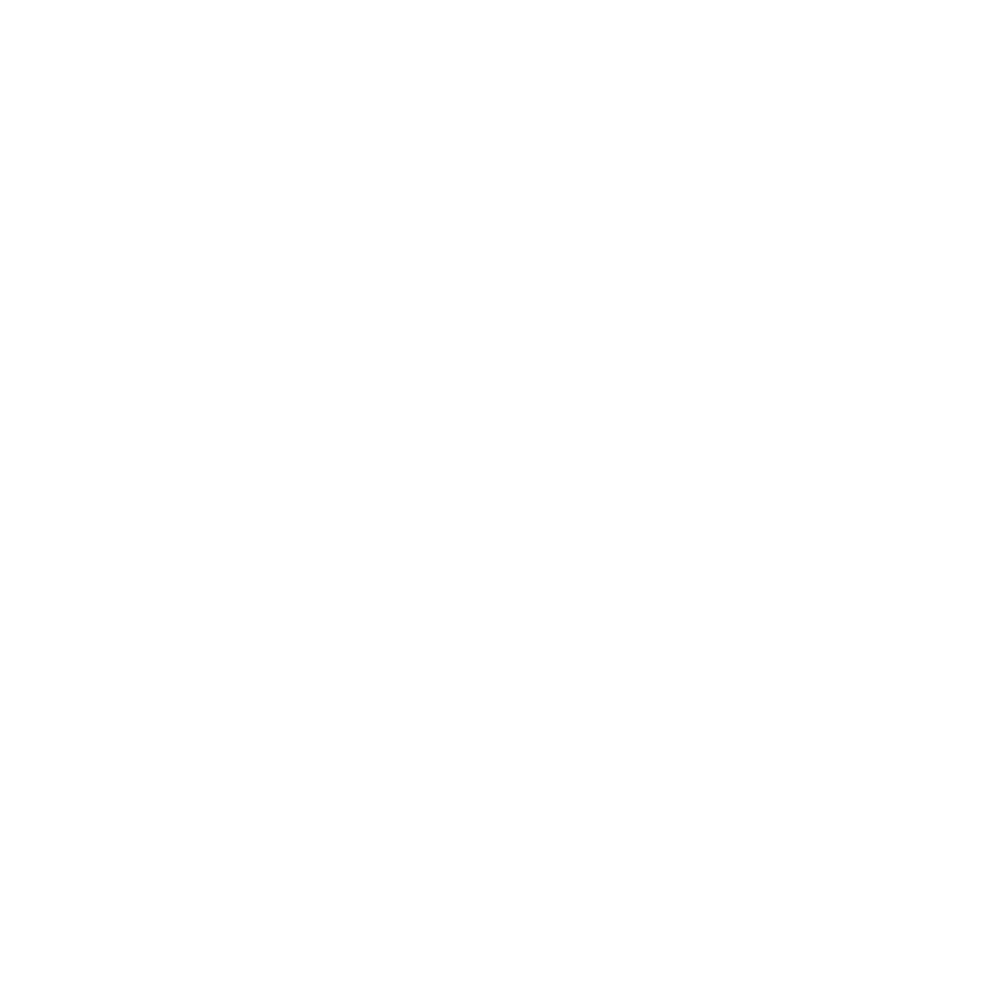
AI big models bring
Storage Challenges
The importance of arithmetic, algorithms, and data in the development of AI has long been known, but storage, as the bearer of data, is often overlooked.
In the process of training AI big models, a large amount of data exchange is required, and storage, as the basic hardware for data, is not just a simple record of data, but is profoundly involved in the whole process of data subsumption, flow, utilization, and other big model training.
If the storage performance is not strong, then it may take a lot of time to complete a training session, which can severely limit the development of large model iterations.
In fact, many enterprises have begun to realize the huge challenges facing storage systems during the development and implementation of large model applications.
From the perspective of the R&D and production process of AI big models, it can be divided into four stages: data acquisition, cleaning, training and application, and each stage puts forward new requirements for storage, for example:
In the data collection session, due to the massive size of raw training data and the variety of sources, organizations want a high-capacity, low-cost, highly reliable data storage base.
At the data cleansing stageThe raw data collected on the network can't be used directly for AI model training, and it needs to be cleaned, de-weighted, filtered, and processed in multiple formats and protocols, which is called "data preprocessing" in the industry.
Compared with traditional unimodal small model training, the amount of training data required for multimodal large models is more than 1,000 times larger, and the preprocessing time for a typical 100TB large model dataset is more than 10 days, which accounts for 30% of the whole process of AI data mining.
At the same time, data preprocessing is accompanied by high concurrency processing, which consumes a huge amount of computing power. This requires that the storage can provide multi-protocol, high-performance support, and complete the cleaning and conversion of massive data in the way of standard files to shorten the duration of data preprocessing.
In the model training session, usually suffer from slow loading of training sets, easy interruptions, and long data recovery times.
Compared to traditional learning models, large model training parameters and training datasets increase exponentially, so how to achieve fast loading of massive small file datasets and reduce GPU waiting time is key.
Currently, mainstream pre-training models have hundreds of billions of parameters, and frequent parameter tuning, network instability, server failures, and many other factors bring about an unstable training process that is prone to interruptions and reworks, requiring a Checkpoints mechanism to ensure that the training is rolled back to the restoration point rather than the initial point.
Currently, Checkpoints require days of recovery time, leading to a steep increase in the overall training cycle for large models, and in the face of the single oversized data volume and future hourly frequency requirements, serious consideration needs to be given to how to reduce the Checkpoints recovery time.
Therefore, whether the storage can read and write checkpoint files quickly also becomes the key to efficiently utilize arithmetic resources and improve training efficiency.
In the application phaseThe storage needs to provide a relatively rich data audit capabilities to meet the demands of the identification of pornography and violence security compliance, to ensure that the content generated by the large model is legal and compliant way to use.
Overall, to maximize the efficiency of AI large model training and reduce unnecessary waste, it is necessary to work on the data. To be precise, innovations must be made in data storage technology.
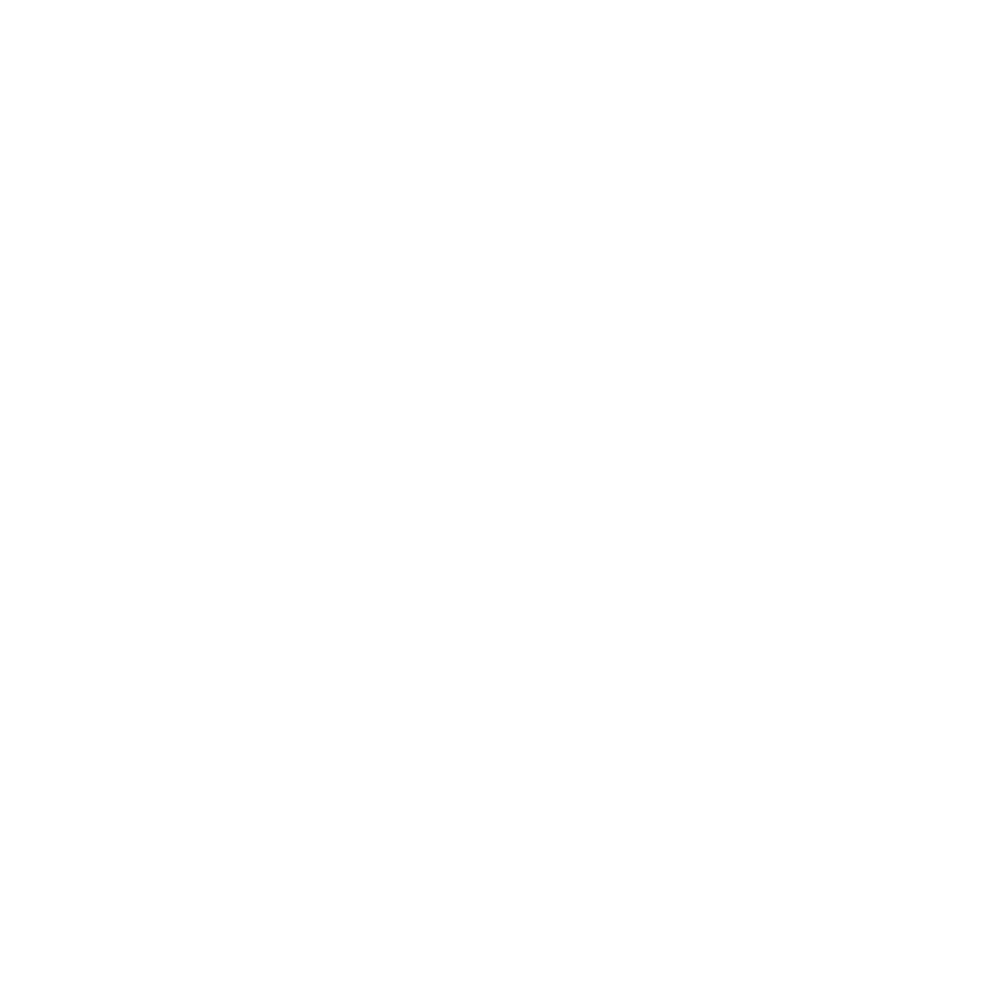
AI forces storage technology innovation
By 2030, the industry is expected to train AI models with 57 times more parameters and 720 times more Token than GPT-3, at a cost of $600,000, down from today's $17 billion, according to the budget of investment firm ARK Invest.
As the price of computing decreases, data will become the main limiting factor in the production of large models.
Faced with the problem of data shackles, many companies have begun to carry out forward-looking layout.
For example, large model companies such as Baichuan Intelligence, Smart Spectrum, and Yuanxiang have adopted Tencent Cloud's AIGC cloud storage solution to improve efficiency.
The data shows that Tencent Cloud's AIGC cloud storage solution can double the efficiency of both data cleansing and training of large models, and halve the time required.
Big model companies and organizations such as KDDI and CAS, on the other hand, have adopted Huawei's AI storage-related products.
The data shows that Huawei OceanStor A310 can realize AI full-process massive data management from data aggregation, preprocessing to model training and inference application, simplifying the data aggregation process, reducing data moving, and improving preprocessing efficiency by 30%.
At present, major domestic vendors have also released storage solutions for AI large model scenarios.
In July 2023, Huawei released two storage products for AI big models - OceanStor A310 Deep Learning Data Lake Storage and FusionCube A3000 Train/Push Hyperconverged All-in-One.
At the November 2023 Yunqi Conference, AliCloud launched a series of storage product innovations for large model scenarios, empowering AI business with AI technology, helping users manage large-scale multimodal datasets more easily, and improving the efficiency and accuracy of model training and inference.
In December 2023, Baidu Intelligent Cloud released the "Baidu Canghai-Storage" unified technology base, and at the same time comprehensively enhanced for data lake storage and AI storage capabilities.
In April 2024, Tencent Cloud announced a comprehensive upgrade of its cloud storage solution for AIGC scenarios, providing comprehensive and efficient cloud storage support for the entire process of AI large model data collection and cleaning, training, inference, and data governance.
Comprehensive major manufacturers of storage technology innovation, you can find that the direction of the technology is more unified, are based on the whole process of AI large model production and development, targeted performance optimization of storage products.
Tencent Cloud, for example, in the data collection and cleaning process, the first need for storage can support multi-protocol, high performance and large bandwidth.
Therefore, Tencent Cloud Object Storage COS can support a single cluster to manage a storage scale of 100 EB level, provide convenient and efficient data public network access capability, and support a variety of protocols to fully support the collection of massive data at the PB level of large models.
Meanwhile, when data cleansing, big data engines need to read and filter out valid data quickly. Tencent Cloud Object Storage COS improves data access performance through its self-developed data gas pedal GooseFS, realizing a read bandwidth of up to several terabytes of ps, supporting high-speed operation of computation and greatly improving data cleaning efficiency.
During model training sessions, it is often necessary to save the training results every 2-4 hours so that they can be rolled back in case of GPU failure.
Tencent Cloud independently developed parallel file storage CFS Turbo, which is specially optimized for AIGC training scenarios, with a total read/write throughput of TiB/s and metadata performance of up to one million OPS per second, both of which are the first in the industry. 3TB checkpoint write time is shortened from 10 minutes to 10 seconds, which significantly improves the efficiency of large model training.
Large model inference scenarios place higher demands on data security and traceability.
Tencent Cloud Data Vientiane CI provides implicit watermarking of images, AIGC content auditing, intelligent data retrieval MetaInsight and other capabilities to provide strong support for the whole business process of data production from "user input - pre-processing - content Audit - Copyright Protection - Security Distribution - Information Retrieval" to provide strong support for the whole process of data production, optimize AIGC content production and management mode, and comply with regulatory guidance. optimize AIGC's content production and management mode, comply with regulatory guidance, and broaden storage boundaries.
At the same time, as training data and inference data grow, there is a need to provide low-cost storage capabilities and reduce storage overhead. Tencent Cloud object storage service provides up to 12 9s data persistence and 99.995% data availability, which can provide continuously available storage services for business.
Overall, as AI big models advance, new trends in data storage are emerging. The market is eager for higher-performance, high-capacity, and low-cost storage products, and to accelerate the convergence and efficiency of all aspects of the big model.
The major vendors are also through technological innovation to continue to meet the needs of the various aspects of the big model, for the enterprise to implement the big model to lower the threshold.
Storage innovation is on the way, forced by the big AI models.
The above content are reproduced from the Internet, does not represent the position of AptosNews, is not investment advice, investment risk, the market need to be cautious, in case of infringement, please contact the administrator to delete.